At Dyno, we believe in collective innovation on our journey of empowering a diverse team of the best problem solvers to drive cutting edge science towards improving patient health. We’re proud to bring together minds from science, technology and business to propel advancements in gene therapy delivery. Our collaborative approach is not only limited to capsids but also within our science and engineering progress. Today, we are excited to share a library for easy workflow construction and submission that we have developed internally and found useful more broadly.
Introduction
Rapid experimentation, scalable machine learning, and computational biology workflows are core to Dyno’s capacity to engineer capsids. Dyno’s engineering team is responsible for building the infrastructure and tooling required to solve all three of these problems. A key component of this is being able to execute computational workflows on demand.
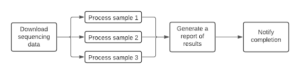
Executing such workflows, particularly larger ones with more steps, requires infrastructure that can flexibly scale in proportion to demand. The rest of this blog post discusses our journey towards building a framework, Hera, which enables fast, scalable remote execution of computational workflows.
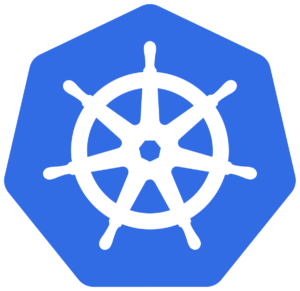
From the beginning, we knew we wanted to use Kubernetes for applications that run in Docker containers. Containers are software environments that isolate the dependencies of Dyno’s applications into entities that are easily deployable to different kinds of environments.
Kubernetes is a container orchestration platform that can scale containerized applications to thousands of nodes, potentially running in multiple availability zones, with a mix of compute resources (e.g NVIDIA K80, T4, or V100 GPUs) easily. While Kubernetes is a flexible way to run containers, it is challenging to write the necessary configurations to execute workflows on Kubernetes directly. Therefore, we sought a higher level framework to enable the execution of tasks in sequence, in parallel, and as dependency graphs.
The search for a workflow engine is never an easy task. We evaluated several workflow engines, such as Airflow. For our base framework, we ultimately landed on Argo Workflows, in large part because we wanted something that was Kubernetes native.

Argo Workflows is an open source framework for orchestrating multi-step workflows in parallel on top of Kubernetes. At Dyno, we decided to adopt Argo mainly for its Kubernetes-native characteristics and its container-focused design. This means that we can take advantage of all of the features of Kubernetes and its integrations into the Google Compute Engine ecosystem, providing us access to resources to run Dyno’s specialized machine learning and computational biology containers!
However, as we discuss more in the Journey to Hera section, neither raw Argo Workflows nor the existing frameworks on top of it really served our needs. Raw Argo was powerful but required specifying workflows in YAML rather than Python. And the existing libraries built on top of Argo were a combination of unintuitive, hard to debug, and broken Python abstractions. So, despite being wary of not-invented-here syndrome, we decided to build our own simpler and more accessible solution.
Hera
The Argo was constructed by the shipwright Argus, and its crew were specially protected by the goddess Hera.
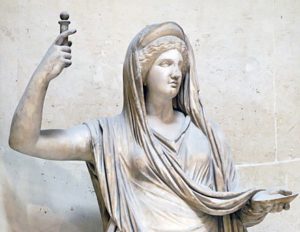
To facilitate adoption of Argo Workflows and transition more of Dyno’s scientific workflows to Kubernetes, we created Hera! Hera is a simple Argo Workflows Python SDK that aims to make workflow construction and submission easy and accessible to everyone! Hera abstracts away workflow setup details while still maintaining a consistent vocabulary with Argo Workflows. For example, Hera parallels Argo in its usage of terms such as “workflow” and “task” to stay consistent with terminology of the Argo UI. This enables users to continue to take advantage of Argo features such as the Argo UI for monitoring execution and logging.
Python functions are first class citizens in Hera – they are the atomic units (execution payload) that are submitted for remote execution. The framework makes it easy to wrap execution payloads into Argo Workflow tasks, set dependencies, resources, etc. Putting Python functions front-and-center allows developers to focus on their business logic to be executed remotely rather than workflow setup. The Github repository provides a concrete example illustrating Hera’s intuitive specification language relative to other frameworks. By comparison to a library such as Couler and the Argo Python DSL, Hera significantly reduces the amount of code you have to write while allowing the user to focus almost exclusively on specifying their logic in vanilla Python!
Hera in detail
First, we start with an example of a workflow that processes a diamond shaped graph, with parallel steps for each task:
from hera.task import Task
from hera.workflow import Workflow
from hera.workflow_service import WorkflowService
def say(msg: str):
"""This can be any Python code you want!
As long as your container satisfies the dependencies :)
"""
print(msg)
# 'my-argo-server.com' is the domain of your Argo server
# and 'my-auth-token' is your Bearer authentication token!
ws = WorkflowService('my-argo-server.com', 'my-auth-token')
w = Workflow('parallel-diamonds', ws)
a = Task('A', say, [{'msg': 'This is task A!'}])
b = Task('B', say, [{'msg': 'This is task B!'}])
c = Task('C', say, [{'msg': 'This is task C!'}])
d = Task('D', say, [{'msg': 'This is task D!'}])
a.next(b).next(d) # `a >> b >> d` does the same thing!
a.next(c).next(d) # `a >> c >> d` also!
w.add_tasks(a, b, c, d)
w.submit()
Notice how much simpler it is to write this workflow in Hera relative to the Argo Python DSL or Couler.Users only need to specify the name of a task, the Python function they want to run, and the specific input the function should receive. Task chaining is also easy and intuitive but also supports arbitrarily complex dependencies specified as code.
Internally, Hera uses the auto-generated OpenAPI Argo Python SDK. There are higher level, strongly typed objects written using Pydantic, such as Task and Workflow, which wrap Argo specific objects, but offer high level interfaces for easy specification. In addition, there are resource objects such as Volume, ExistingVolume, and Resources, that can be stitched together to construct the resources of a Task. For example, a Resources object can be constructed to dictate that a task needs to use 4 CPUs, 16Gi of RAM, 1 NVIDIA K80 GPU, and a volume of 50Gi. This can be then passed to a Task, then submitted as part of a workflow. By default, tasks are assigned 1 CPU and 4Gi of RAM.
from hera.resources import Resources
from hera.task import Task
from hera.volume import Volume
from hera.workflow import Workflow
from hera.workflow_service import WorkflowService
def do():
import os
# will print a list where /vol will have 50Gi!
print(f'This is a task that requires a lot of storage! '
'Available storage:\n{os.popen("df -h").read()}')
# TODO: replace the domain and token with your own
ws = WorkflowService('my-argo-server.com', 'my-auth-token')
w = Workflow('volume-provision', ws)
d = Task('do', do, resources=Resources(volume=Volume(size='50Gi', mount_path='/vol')))
w.add_task(d)
w.submit()
Journey to Hera
Raw Argo Workflows
As mentioned already, we started our journey to Hera using Argo Workflows directly. Initially, the introduction of Argo Workflows to Dyno led to a massive increase in the scalability of computational biology and machine learning jobs! Whereas previously, we’d been stuck waiting for sequential processes to execute in Jupyter notebooks, or independent VMs, using Argo enabled us to regularly run jobs across thousands of containers on Kubernetes. These jobs range from processing raw, sequencing data, training hundreds of machine learning models in parallel on single or multiple GPUs, and executing regular cron jobs for infrastructure maintenance.
However, Argo’s introduction to Dyno came with its own set of challenges. Argo Workflows are typically configured through YAML files, which have a particular syntax for structuring workflow templates, steps, graphs, parallelism, etc, which is just as cumbersome as managing Kubernetes. We mostly use Python at Dyno so we wanted a Python library for scheduling Argo workflows.
Argo Python DSL
Our first Argo workflow framework was a library called the Argo Python DSL, a now archived repository that is part of Argo Labs. While it allowed Dyno to extract immediate value out of Argo Workflows, it came with several challenges. First, we (the engineering team) struggled to motivate Aaviators outside of Engineering to use it… for good reasons! The DSL’s abstractions mostly mirrored Argo’s itself, requiring users to understand low level Argo Workflows concepts such as V1Container, or V1alpha1Parameter. Rather than understand these Argo-specific concepts, scientists want to focus on specifying workflow tasks and their dependencies, and the logic of a machine learning or computational biology experiment. The overall consequences of surfacing these Argo specific concepts results in a significant increase in workflow setup complexity, as illustrated by the following example:
class DagDiamond(Workflow):
@task
@parameter(name="message", value="A")
def A(self, message: V1alpha1Parameter) -> V1alpha1Template:
return self.echo(message=message)
@task
@parameter(name="message", value="B")
@dependencies(["A"])
def B(self, message: V1alpha1Parameter) -> V1alpha1Template:
return self.echo(message=message)
The DSL also had other quirks that confused non-expert users. As an example, obtaining parameter values to a function used as a step in workflow uses core Argo Workflows syntax specified inside a string such as {{inputs.parameters.message}} when using V1alpha1ScriptTemplate, which allows users to submit code scripts. Not only is this challenging to remember but it also breaks type checking and any other error-preventing tooling.
For these and other reasons, we eventually started looking into alternatives to the DSL that would make developing workflows easier for scientific users.
Couler
The second library that Dyno tried for Argo workflow construction and submission was Couler, which is an active project that aims to provide a unified interface for Argo Workflows, Tekton Pipelines, and Apache Airflow (although they currently only support Argo Workflows). While useful, it still posed challenges because of the graph task dependency interface – it is hard to set up a chain of tasks to be executed as part of a graph. There are several Couler abstractions, such as dag, that impose requirements like the use of lambda functions in Python, which make it challenging to understand workflow setup and dependencies:
def diamond():
couler.dag(
[
[lambda: job(name="A")],
[lambda: job(name="A"), lambda: job(name="B")], # A -> B
[lambda: job(name="A"), lambda: job(name="C")], # A -> C
[lambda: job(name="B"), lambda: job(name="D")], # B -> D
[lambda: job(name="C"), lambda: job(name="D")], # C -> D
])
Notice how job A has to be specified multiple times in order to set up the dependency for job B and C. In addition, notice how one has to set up lists for the Couler dag in a specific structure, which can further confuse users. Another problem we have encountered with Couler is the absence of a workflow submitter object that would allow Dyno to submit workflows to the Argo server accessible at a specific internal domain. By comparison, Couler requires users to have a Kubernetes configuration, typically located at ~/.kube/config, so workflow submissions can occur through the Argo Kubernetes deployment (accessed via kubectl). From an authentication and user experience standpoint, this was a “no go” for Dyno from the start – we do not want our scientists to face the need to learn Kubernetes concepts, the use of kubectl, or gcloud for performing context switching between different Google Cloud projects. What we needed was an easy way to submit workflows, perform authentication with a server, and abstract away complexity associated with the use of Argo objects.
Conclusion
Hera makes Argo Workflow construction and submission much more accessible. Given the internal success we’ve had with Hera, we decided to open-source the library. In collaboration with the core maintainers of Argo Workflows (Alex Collins, Saravanan Balasubramanian, Yuan Tang, and Jesse Suen), Dyno released Hera under Argo Labs. You can also watch the initial release presentation on the Argo Workflows Community Meeting from October 2021.
If you’d like to work on infrastructure challenges such as supporting highly scalable machine learning and computational biology workflows on a variety of resources, support the open-source development of Hera and Argo Workflows, and contribute to modernizing the biotech application tech stack via the introduction of DevOps principles into scientific pursuits, check out our Careers page. We are always on the lookout for great engineers!
Dyno Therapeutics
Flaviu Vadan
Senior Software Engineer
Dyno Therapeutics
Stephen Malina
Machine Learning Scientist